“There‘s no consciousness, no intelligence,” says Peter Freudling, researcher and developer at the Ars Electronica Futurelab. He‘s talking about machines, algorithms, and systems that are already making all kinds of decisions easier for us, and in some cases even taking them off our hands. The conversation is about a technology that is getting all the attention, is with us every day, and is set to take on an even larger role in the next years and decades. But according to Peter, it‘s not actually that intelligent.
So how does artificial intelligence actually work? What’s behind it? And how can you explain that to a museum visitor in a comprehensible way? That‘s what Peter Freudling and his colleagues Ali Nikrang and Stefan Mittlböck-Jungwirth-Fohringer have been working on intensively for the past half year. For the new exhibition “Understanding AI” at the Ars Electronica Center, they developed art and science installations designed to make AI more comprehensible – places for observing, participating, and trying it out.
Ali Nikrang explained in part one of our interview series what actually lies behind the AI technology. Here, he joins Peter Freudling and Stefan Mittlböck-Jungwirth-Fohringer to explain how specially designed installations allow people to experience AI in “Understanding AI.”
In the first part of the interview, we already learned a lot about artificial intelligence. How do you approach such a complex topic?
Stefan Mittlböck-Jungwirth-Fohringer: Our primary task was to create didactic installations that explain artificial intelligence.
Peter Freudling: We wanted to demystify the technology because currently that term is a major buzzword and so it‘s often used incorrectly. Ultimately, it‘s really just about algorithms that are trained by people – there‘s no consciousness, no intelligence in a true sense.
Stefan Mittlböck-Jungwirth-Fohringer: Artificial intelligence is based on simple mathematical functions and formulas. No more and no less. We want to make visitors aware of what‘s really behind the technology and the images we have of it. A foundational understanding.
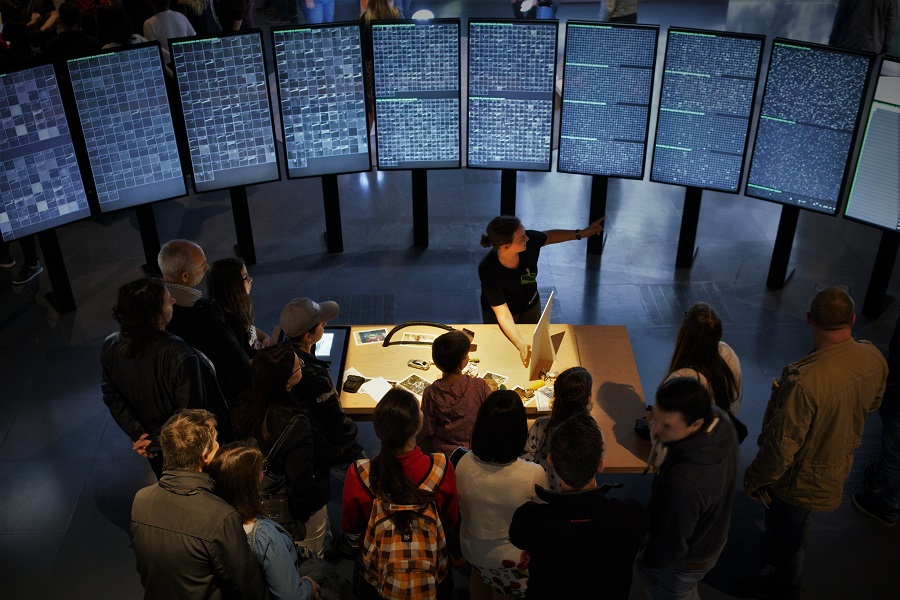
Credit: Ars Electronica / Robert Bauernhansl
You created several installations for this purpose. Can you describe the things you worked on that are now on display at the Ars Electronica Center?
Stefan Mittlböck-Jungwirth-Fohringer: The installation that took the most work was the Neural Network Training. In that one we are trying to explain the artificial neuron with practical examples and in a playful way. To do that, we developed two examples: in one, you train a neural network on the right color combination of writing and background, in the second one you train a network to recognize which lifeforms are dangerous for a mouse.
Peter Freudling: Here we want to emphasize above all that it‘s in our power to decide what is right and wrong. What’s dangerous? What’s harmless? The software answers according to its training – it doesn’t matter what would actually be right or wrong. Neural networks are nothing more than an accumulation of many switches that are constantly resetting themselves – just like our brain. With us, synapses form, and something similar happens between artificial neurons.
Stefan Mittlböck-Jungwirth-Fohringer: As a visitor, you can set the input values in a neuron of this kind and then observe what happens. The neural network produces a statistic of all the values that have been entered, a probability. In the color example it’s just like that: I, the visitor, select which background color and which color of writing are the most readable for me – I train the network and do it several times in a row. From a certain point onwards, the network is done training and can recognize independently which color combinations are easy to read and which are not.
So here the human aspect is emphasized again – the way you train an artificial intelligence system determines the answers it will give.
Stefan Mittlböck-Jungwirth-Fohringer: Exactly. That highlights the moral questions behind this technology: who’s training the network? With what background? With what intention?
Peter Freudling: We at least hope these questions will open up.
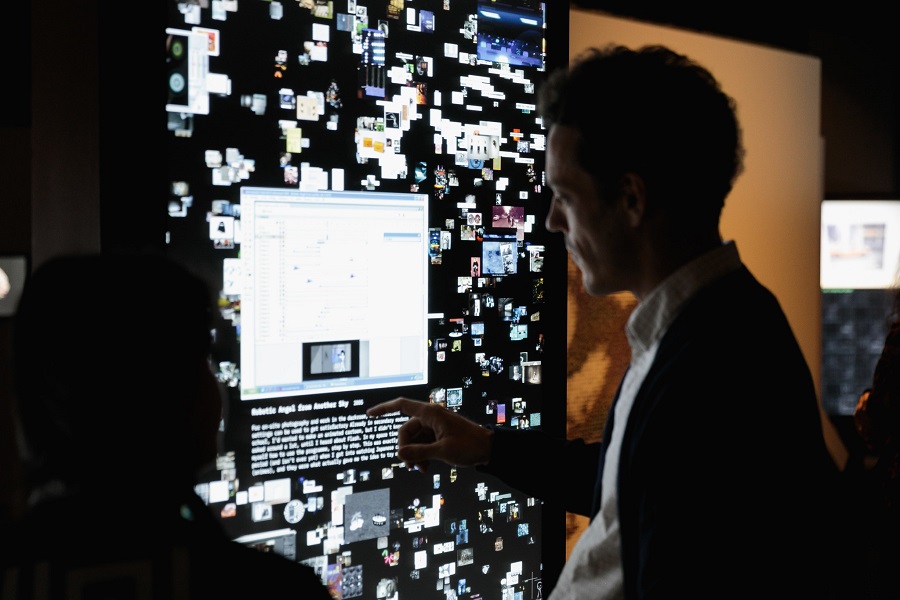
Vector Space. Credit: vog.photo
After an installation that shows examples of text written by a machine, the visitors see an installation caleld Vector Space.
Ali Nikrang: In this installation you can organize Prix Ars Electronica winners from the past few decades. Objects that are similar are supposed to be shown together. However, what defines this similarity can be selected: it might depend purely on the picture, or purely on the accompanying text, or on a combination of the two. For this we use the same model that is used in image recognition. However, what‘s interesting for me is not the task itself, but what you learn from the result.
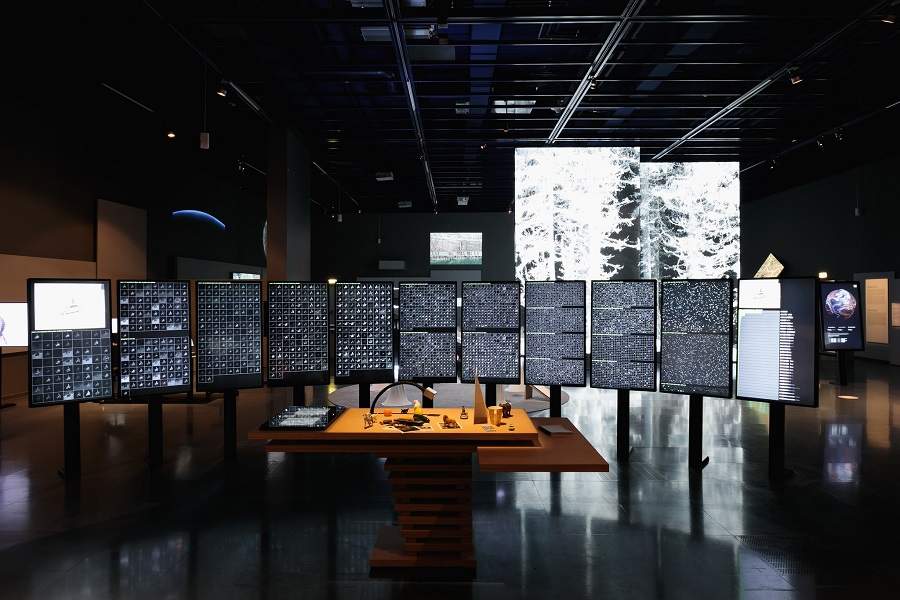
Credit: vog.photo
You see that it’s really just about analyzing data.
Peter Freudling: If you imagine our entire archive, without tags or order – it’s impressive that it can be put in order so quickly. It suddenly becomes searchable, things are grouped together that the model thinks belong together.
Ali Nikrang: You can discover new relationships. It‘s navigating out of curiosity.
Stefan Mittlböck-Jungwirth-Fohringer: What I find exciting about the project is this basic understanding of multidimensional vector spaces.
Ali Nikrang: That‘s why it’s called Vector Space. It’s just like in language recognition, you have different terms in a virtual space with many dimensions. The word “blue” is located somewhere, just like the word “red.” Probably they are close to each other, they’re both colors. “Project” and “undertaking” are probably also close together because they have the same meaning. This vector space is multidimensional, every word has hundreds of dimensions that are described with hundreds of numbers.
Peter Freudling: These vector spaces try to represent our language. For example, “sun” has something to do with planets, but can also be in the weather category. The data in this installation are arranged according to these vectors as well – based on similarity of images or text.
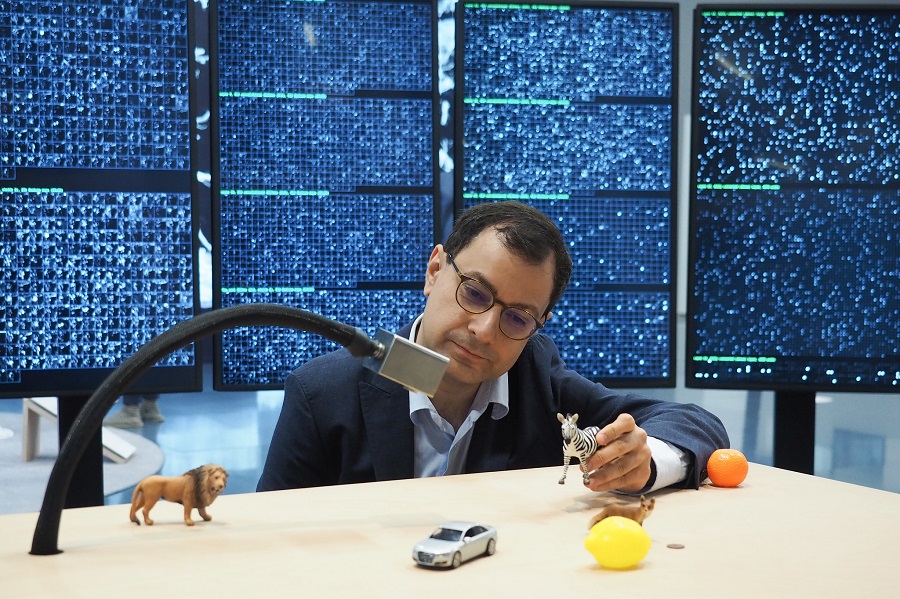
Ali Nikrang. Credit: vog.photo
At the end of the didactic course there are 11 screens. What’s that all about?
Ali Nikrang: Here we see the core technology of deep learning, a convolutional neural network. The installation visualizes how image recognition works: you hold an object in front of the camera and the system tells you what it is. It was trained with a thousand images per category. In order to show how something like that works, we visualized the process on 11 screens. You see the activations – that’s what they’re called. In deep learning, there are different layers, which you can see here. At the beginning, the system only recognizes primitive elements, lines or curves , deeper in the network these elements combine into larger objects. However, the information also becomes more and more abstract, no longer comprehensible to us humans. On the other hand, the result is very clear to the machine.
Stefan Mittlböck-Jungwirth-Fohringer: We wanted to be able to watch a neural network analyzing in real time, so to speak. We say “the machine sees,” but it’s actually a matter of filters, which you can see very well here.
Then with Comment AI, we go back to text…
Peter Freudling: So far, most of the models in the field of language recognition or production are in English. Here we use a data set of posts from the website standard.at, ranging from neutral to hate speech, there is really a bit of everything here. Visitors can assign these posts to categories and train the network that way. So, soon there will be a data set of German-language posts which, for example, could be made available to university institutes.
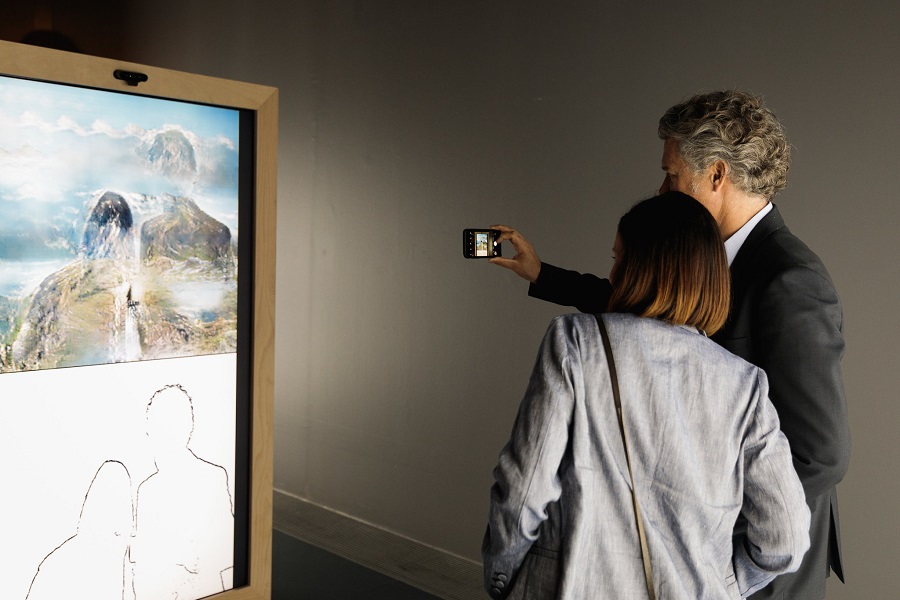
Credit: vog.photo
The installation ShadowGAN goes in a very different direction – could you tell me a bit about that?
Stefan Mittlböck-Jungwirth-Fohringer: Here you stand in front of a white wall, are captured by a camera, and finally represented in summer or winter landscapes. We wanted to create an awareness of the fact that when a neural network has only learned certain data sets, that’s all it can show. It’s fun for the visitors, they get to see themselves as a landscape!
Ali Nikrang: The installation works with a generative adversarial neural network or GAN. You train two models against each other, a faker and a judge. There is also a data set that is already marked as correct. The judge receives random images from the data set and from the faker, and has to be able to tell the difference and decide which image comes from where. The faker is trained to create better and better pictures that the judge cannot recognize as forgeries.
What I find very interesting about that is that the system learns the concept of something. Here, it knows the concept of summer or winter – there’s snow at the bottom, sky at the top, that’s winter.
Stefan Mittlböck-Jungwirth-Fohringer: At our installation GANgadse right next to this one, it’s the same except that the system here was only trained with cats. People draw outlines and the machine automatically fills them in with catlike elements. It doesn‘t matter what you draw – it will always be a cat.
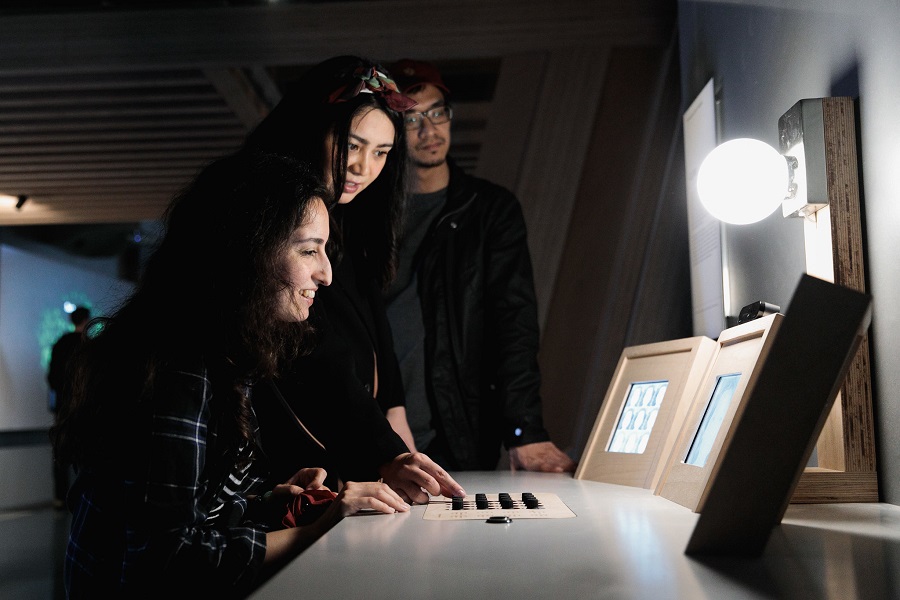
Glow / Glow Diederik P. Kingma, Prafulla Dhariwal; OpenAI. Credit: vog.photo
That is also one of the core problematic elements of the installation GLOW. Here you can change your own appearance…
Ali Nikrang: The model of OpenAI was trained with lots of faces from Hollywood stars, we designed the interface for that. When I sit in front of the installation, I can make myself more or less attractive, put on glasses or not, etc. Here, the system has also learned the concept that a pair of glasses, for example, always covers both eyes. However, that also means that if you are a woman and you sit in front of the system and want to give yourself a beard, it will be difficult. It will only work if you turn up the “manliness” factor. The system has learned it that way. The same goes for makeup and men – but what does make-up have to do with gender?
Stefan Mittlböck-Jungwirth-Fohringer: Artificially intelligent systems are just as racist and misogynist as the people who train them. That is exactly the discussion we wanted to encourage.
Peter Freudling: What I think is important about these systems can be seen above all in the convolutional neural network: it always recognizes all the thousand categories in one object, just with lower or higher probability. It’s not consciousness, just a tendency. Making a decision based on that will still be up to us.
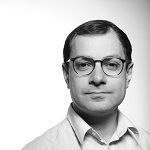
Ali Nikrang is a senior researcher & artist at the Ars Electronica Futurelab, where he’s a member of the Virtual Environments research group. He studied computer science at Johannes Kepler University in Linz and classical music at the Mozarteum in Salzburg. Before joining Ars Electronica’s staff in 2011, he worked as a researcher at the Austrian Research Institute for Artificial Intelligence, where he gained experience in the field of serious games and simulated worlds.
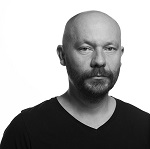
Stefan Mittlböck-Jungwirth-Fohringer is a lead producer and artist at the Ars Electronica Futurelab. He studied visual arts and cultural studies in Linz Art University’s Painting and Graphics program, and has been a PhD candidate under the tutelage of Prof. Karin Bruns and Prof. Thomas Macho since 2012. He has worked at the Ars Electronica Futurelab since 2001, and has been a member of the MAERZ artists’ association since 2006.
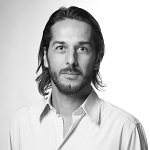
Peter Freudling is Lead Producer an Artist at the Ars Electronica Futurelab.
You can have a look at these installations within the exhibition “Understanding AI” at the Ars Electronica Center right now – and of course during the Ars Electronica Festival (5 – 9 September, 2019), too! To learn more about Ars Electronica, follow us on Facebook, Twitter, Instagram et al., subscribe to our newsletter, and check us out online at https://ars.electronica.art/news/en/.