You get a weird feeling viewing Ali Nikrang’s “Style Video” in Deep Space 8K—it’s as if you were standing amidst a painting by Roy Lichtenstein or Wassily Kandinsky. The stereoscopic video is based on a film capturing a downhill run on the Streif in Kitzbühel, perhaps the world’s most famous ski slope. Thanks to artificial intelligence, this wild ride looks like it was painted.
Ali Nikrang, an artist and researcher at the Ars Electronica Futurelab, tells us how it works in this interview. At the Ars Electronica Festival September 6-10, 2018, you can experience this and other works, and attend discussions on the subject of deep learning at “Creative AI – Style Transfer” in Deep Space 8K at the Ars Electronica Center.
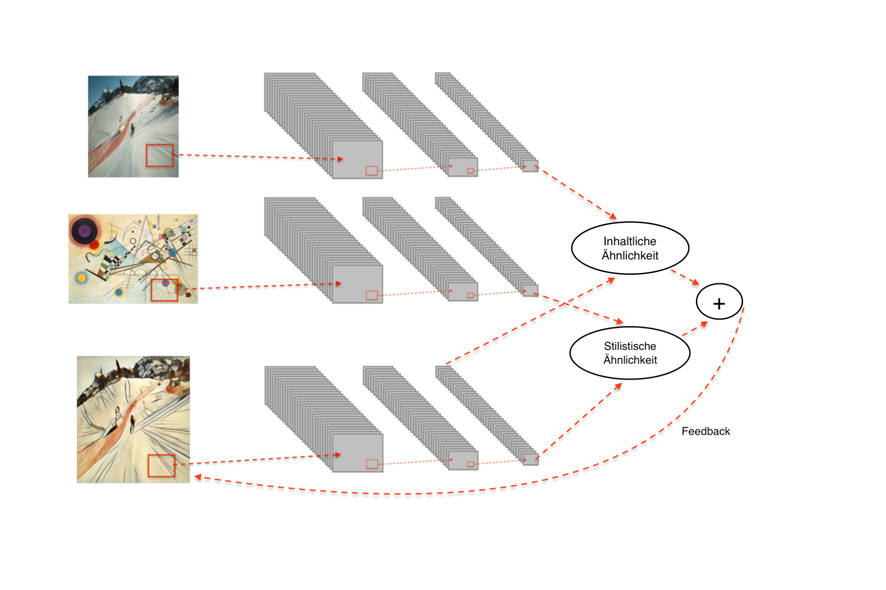
Credit: Ali Nikrang
What’s the story with the title “Creative AI – Style Transfer”?
Ali Nikrang: The centerpiece of our presentation in Deep Space 8K is a video that attempts to imitate the style of a painting or picture. In this project, we used a stereoscopic video shot on the Streif in Kitzbühel, one of the world’s most difficult and dangerous downhill runs. Style transfer, a technique from the field of deep learning, immerses the viewer into the forms and colors of artists such as Kandinsky and Lichtenstein, and makes the Streif look like a three-dimensional painting in Deep Space 8K. The extraordinary thing about it is that you get the feeling you’re right in the middle of a painting—and in stereo, no less—even thought the actual images weren’t stereoscopic.
Are there particular styles that are especially well suited to Style Transfer?
Ali Nikrang: Yes, there are. It’s interesting that the more realistic a style is, the worse the results are. That means that when you take a painting by, say, Da Vinci or a photorealistic painter, it’s more difficult. For this project, we experimented with a great many things, even photos, and, of course, this yields interesting outcomes. But for us, it’s not enough when a frame looks good; needless to say, we want the whole video to be good. We ultimately indentified three styles that are very well suited, divided the Streif video into three parts, and let it rip. For the sake of completeness, there are models that have been designed for photorealistic styles, for example, to combine the style of one photo with the content of another, but our goal was to produce a more abstract and picturesque result.
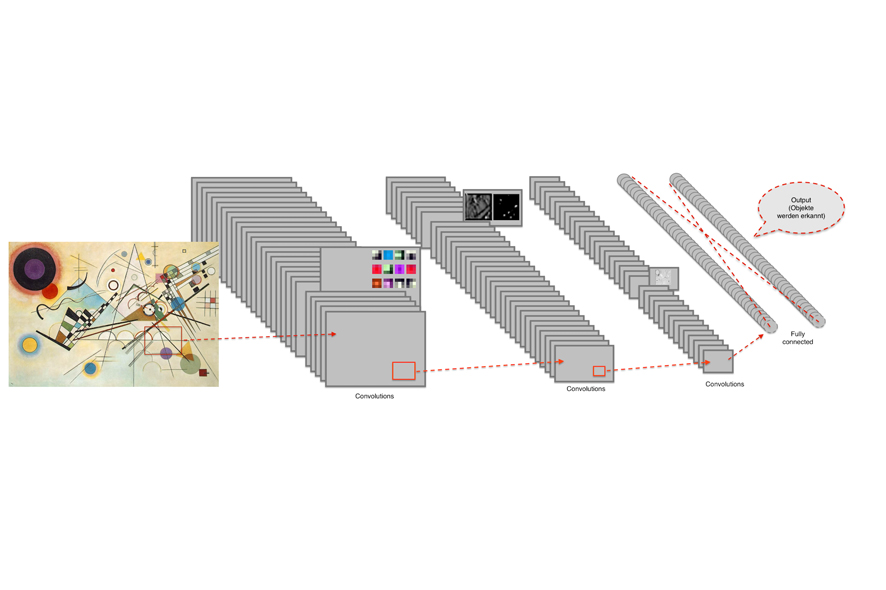
Credit: Ali Nikrang
Why do you suppose more realistic images don’t work as well?
Ali Nikrang: It has to do with the nature of this technique. So this brings us to how this actually works. As I mentioned, style transfer comes from deep learning. Deep learning is applied in many different areas, one of the most important of which is the visual perception of the world. In principle, objects in the real world are classified. In order for computers to recognize the objects, the computers have to understand or recognize the characteristics of the visual world—for example, what constitutes a cat?
The computer recognizes a cat as a combination of pixels. From these pixels, it has to recognize various traits—there are certain forms for the ears; particular colors; it has to see two eyes, etc. Thus, it can’t just think on the pixel level; it has to be able to assemble various features. And that’s why it’s called deep learning—it’s a learning process that entails several levels. The first levels are very primitive, since this really is a matter of a group of a few pixels. The deeper you go, the more these levels are combined until you ultimately have a large combination of various layers.
Style transfer is a byproduct of this technique in which you try to use two images to generate a third one, whereby the typical characteristics of one image are applied to the second one.
So it’s a mixture of these two images or styles.
Ali Nikrang: Exactly. You input two images—a style image and a content image. And from them, you want to generate a third image that applies the typical characteristics of the style image to the content image, whereby the content image should be recognizable as such but display the typical traits of the style image. So how do you do that? This is where the technique of deep learning comes into play. The typical traits of a style image can be seen in the middle levels of a deep learning network. These can be interpreted like an artist’s brushstrokes. These middle levels are then combined with the last levels of the content image, where very abstract information about the structure of the image is hidden. This maximizes the results’ similarities to the last level of the content image and to the middle levels of the style image.
How does a computer learn to correctly identify these traits?
Ali Nikrang: A probability is computed. The results are based on a training phase in which you have a lot of pictures but you always know the answer. For instance, you show the image of a cat and let the computer identify the cat. In the beginning, some sort of result will come out of this, but because the answer is know, “wrong answer” is the feedback received. So you go back and find out which parameter or which filter led to the wrong result, until, after millions of images, the computer finally recognizes a cat as such.
This feedback takes place automatically?
Ali Nikrang: Yes. Because a trained model is very big, it can also be downloaded from companies like Google. These companies have huge computing facilities, but even they have to train for weeks. When you then download the model, it no longer has to work with the initial images. The network has learned the typical characteristics of the visual world, but you can supplement them with your own images. Of course, it recognizes only those objects it has learned—if, for example, there’s a class “cat” but no category “tiger,” then the computer will probably classify a tiger as a cat. But the great thing about the model is that you don’t have to train the whole network; you can download it and add an additional level. This can be done even with a normal computer.
How do you use the neural network for style transfer?
Ali Nikrang: In style transfer, we also have to calculate and compute, of course, but the task isn’t to classify objects. Style transfer entails three networks—one for the style image, one for the content image, and a third one. At the outset, the third one pure noise; it’s assembled from the middle levels of the style image and the final levels of the content image.
How long does it take until a entire styled video emerges?
Ali Nikrang: It depends on which model you take. There are different options—some are faster than others. It varies from several hours to several days, depending on the model.
One problem we had with this project was that style transfer works better when we first scale down the video a bit. Plus, we want to screen the results in Deep Space 8K, which is also somewhat problematic because it calls for such high resolution. So we ultimately decided to use another model from the field of artificial intelligence, one whose task is to scale the images up in a realistic way. So it’s not like using normal visual programs in which the pixels are interpolated; instead, in this case, there really does occur an intelligent process of consideration as to how to increase the resolution so that the image looks as realistic as possible.
The results will be shown at the Ars Electronica Festival in Deep Space 8K. What exactly can festivalgoers look forward to?
Ali Nikrang: At the festival, we’ll view the original video and say a few words about how this actually works. We also want to get into where this technique is headed—I mean, this isn’t going to stop with style transfer. An example of a new task would be to apply the characteristics of summer to winter. And I’ll be screening an example in which the technical assignment is quite similar. Here, the processor has to recognize: What makes the summer summer and the winter winter? This presentation will definitely be of interest to anyone who’s interested in neural networks and deep learning. We won’t only be showing finished works; we’ll also be discussing possibilities. After all, this isn’t the end of the story. There’s a lot of research going on in this area. New results will definitely be emerging, and improved models are coming out by the month.
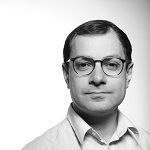
Ali Nikrang is a senior researcher & artist at the Ars Electronica Futurelab, where he’s a member of the Virtual Environments research group. He studied computer science at Johannes Kepler University in Linz and classical music at the Mozarteum in Salzburg. Before joining Ars Electronica’s staff in 2011, he worked as a researcher at the Austrian Research Institute for Artificial Intelligence, where he gained experience in the field of serious games and simulated worlds.
“Creative AI – Style Transfer” will be screened in Deep Space 8K at the Ars Electronica Center during the Ars Electronica Festival September 6-10, 2018.
To learn more about Ars Electronica, follow us on Facebook, Twitter, Instagram et al., subscribe to our newsletter, and check us out online at https://ars.electronica.art/news/en/.